Before discussing the future of data science and big data analytics, let’s consider a scenario. Imagine you’re the head chef at a reputed restaurant that sources ingredients from all over the world. Say, you’ve got baskets full of apricots from Turkey, Kiwi from New Zealand, almonds from California, and bananas from Brazil. Your job will be to decide how to prepare dishes that combine these different varieties the best. From finding out the nutritional value of these fruits to which flavors work well together and what additional ingredients should be added to make the unified dishes more delicious, you’ll have to derive value from these fruits. At the same time, you’ll need to consider how these dishes would influence the restaurant’s menu and reputation.
This analogy would help you to understand how big data and data science work. Just like the different fruits, big data is a large volume of data sets – both structured and unstructured. Thus, big data refers to an immense volume and variety of information assets that are generated rapidly. Similar to the chef’s expertise, data science helps you to process such data to get useful insights for informed decision-making and process automation. The future of both these domains is bright though data science has a clear edge. Perhaps that’s why “how can I become a data scientist?” is a frequently asked question and reputed data science bootcamps in Silicon Valley attract participants galore. If you too have a plan to become a data scientist or work with big data, knowing what the future holds for them would help.
In today’s digital landscape, data has become one of the biggest and most important assets for almost all organizations. Data can be fetched from anywhere and it’s actually transforming the way we live.
If you’re interested in working with data, it’s extremely important to have a clear understanding of the different avenues related to it. In this post, we’ll discuss the differences between data science and big data analytics. Though these terms are interlinked, there’s a huge difference that lies between them almost in every aspect. Let’s start the discussion.
The promising future of big data and data science
From financial services and retailers to media and telecommunications, big data is increasingly used for operational functions, compliance and customer analytics, and fraud prevention. According to IDC, by 2025, it’s expected that 75% of the global population or 6 billion users will be interacting with online data each day. This means in every 18 seconds, each of these connected users will be having one data interaction at the least. As businesses gain the capability to store such huge volumes of data, they’ll need to get them analyzed too to benefit from useful insights. And that’s where data science can help. For instance, if you’re sitting on a pile of data, you can use different types of sophisticated statistical and predictive analysis (think AI or machine learning) to make sense of the structured and unstructured data.
According to the US Bureau of Labor Statistics, 11.5 million jobs in the field of data science and analytics are expected to open up by 2026. With a dearth of data scientists at present, the gap would widen in the future if adequate experienced professionals aren’t trained. This makes data science bootcamps in Silicon Valley an attractive prospect to jumpstart your career in data science.
Data science
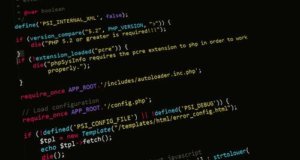
It’s the field that encompasses almost everything related to data – from preparation of data to data cleansing to data analysis, and deals with both structured and unstructured data. Data science can be considered as an umbrella term which includes various scientific methods within its ambit. It combines statistics, mathematics, problem-solving, and much more.
Big data analytics
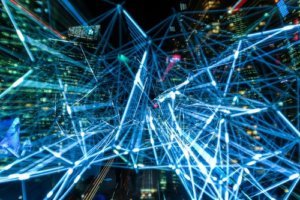
This field involves the application of mechanical or algorithmic processes in order to derive operational insights for complex business solutions. It’s all about examining raw data to support decision making. Big data analytics involves inspecting, transforming, cleansing and modeling data.
Applications:
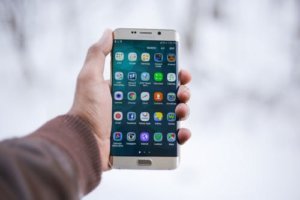
Applications of data science
- Digital advertisement: Data science algorithms are used by the entire digital marketing domain – from digital billboards to display banners.
- Internet search: Data science algorithms are utilized by search engines to offer the best results for search queries within a fraction of seconds.
- Recommender systems: Here, companies use data science technologies to promote their products as well as recommendations according to the relevance of information and the user’s demands. These systems not only enhance user experience but also make it easier to find relevant products or services from billions of them.
Applications of big data analytics
Big data analytics is used in a diverse range of fields. Some of them include:
- Gaming sector: Here, companies use big data analytics to get insights such as likes, dislikes, relationships of the users etc.
- Healthcare sector: Healthcare service providers use big data analytics to perform tasks like tracking and optimizing patient flow, tracking the use of equipment and medicines in the facilities, organizing patient information, etc.
- Travel sector: Travel companies use big data analytics to optimize buying experiences through different channels. They also get consumer preferences and desires, and find correlation between current sales and subsequent browsing, which let them optimize conversions.
Job responsibilities:
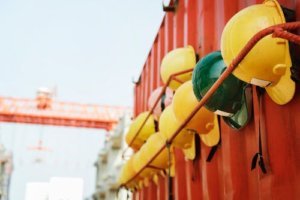
Data science professional
Data science professionals perform an exploratory analysis to obtain insights from data. Different kinds of machine learning algorithms are used to identify the occurrence of a specific event in the future. They focus on identifying unknown correlations, hidden patterns, and market trends, among others.
Big data analytics professional
The responsibilities of big data analytics include dealing with a large amount of heterogeneous data captured from different sources and arriving at a high velocity. These professionals describe the behavior and structure of big data solutions and how they can be delivered utilizing big data technologies like Spark, Hadoop etc based on the requirements.
Skill sets required:
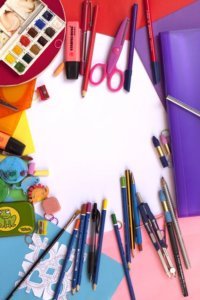
Data science professional
- To become a data science professional, you should have excellent analytical skills together with excellent data management skills. A large percentage of data science professionals hold a Master’s degree or a Ph.D. degree with exceptional skills in statistics, programming, and mathematics.
- Programming skills required include C/C++, Python, R, SAS, Pearl, Java languages etc.
- Require technical skills include machine learning tools, data mining, data managing skills, unstructured data techniques etc.
- Sound knowledge of database systems together with essential business skills like communication, industry knowledge etc.
Big data analytics professional
- As an aspiring big data analytics professional, you’d need to have a robust understanding of programming languages like R and Python.
- You should have excellent skills in statistics and mathematics.
- Data wrangling skills are also extremely important to map out and convert data into another format.
- Other required skills include data visualization, machine learning skills, and communication skills.
Pay packages:
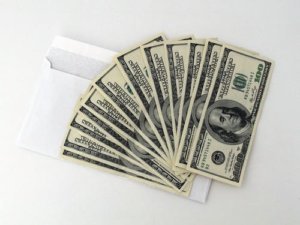
Though both the professionals work in the same domain, the salaries earned by a data science professional and a big data analytics professional vary to a good extent.
The average salary of a data science professional can be around $113, 436 per year, whereas a big data analytics professional can expect to earn around $66,000 per year.
The career path:
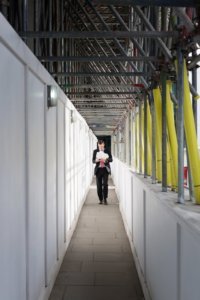
If you’re new to the field of data, data science and big data analytics may seem something that’s interchangeable, but they’re different in reality and so are their career paths. Let’s have a look at them.
Data science professional
Given the huge amount of data being churned out through different devices throughout the world every day, organizations have become highly interested in gleaning valuable insights from their data collection processes. Here’s the ideal path that you can take to become a data science professional.
- You should get accustomed with the most commonly used programming languages used in the field like R, Python, and Java, and refresh your knowledge in statistics and applied mathematics. It’ll also help to understand whether the role of a data science professional is the right fit for you.
- The most preferred majors in data science are mathematics, statistics, information technologies, computer science etc. Having a good understanding in any or a couple of these fields would help you to stay a step ahead of your competition. You should also continue to learn database architecture, programming languages etc.
- Try to start developing your professional networks by building and nurturing connections with different communities, joining internship opportunities etc.
- There’re many organizations that offer entry-level jobs in data science. Look for positions like Junior Data Scientist or Junior Data Analyst. Try to obtain some system-specific certifications or training in data-related fields (for example data visualization software) as they might help you to get an entry-level job faster.
- If you want to make your career opportunities relatively higher, try to obtain an advanced degree like a Ph.D. degree or a Master’s degree.
If you want to get promoted fast or become a data science professional who’s in high demand, try to obtain additional experience. Remember that businesses value results. So, having leadership and project management experience coupled with strong technical skills will help you get more significant opportunities.
Big data analytics professional
Your key responsibilities will include understanding the insights and trends, which are revealed by the huge datasets. Let’s have a look at how you can become a big data analytics professional.
- Consider studying data analysis or applied statistics together with computer science classes that focus on database management and project management.
- It can be a little difficult to gain direct employment as a big data analytics professional without any experience. So, try to take up internship offers to gain valuable experience together with some relevant training that would facilitate additional skill development.
- Try to get an entry-level job like a statistical assistant to get valuable experience and on-the-job training.
- Consider attending in-house training classes as much as you can, particularly those about big data management and analytical software programs. They’ll help you to get crucial knowledge and experience, which in turn would let you reach the level you desire.
- An advanced degree will bring you more job opportunities and thus, help you proceed further in your career. The benefits of training employees in cutting-edge tools and technologies, including obtaining a Master’s degree in big data management, data analytics, or data science, can provide businesses with the necessary expertise and knowledge to succeed in today’s data-driven world. Also, there’re some online academies that offer valuable certifications that will help you gain valuable real-world experience. And the best part about these certifications is that you can continue studying while maintaining your job.
One major thing to remember
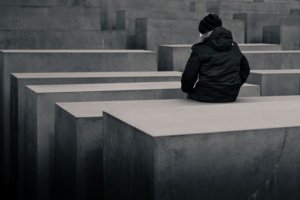
Regardless of whether you choose to become a data science professional or a big data analytics professional, you’ve to stay relevant in your domain. In today’s age of continual technological innovation, continuous education has become more crucial than ever. A career-oriented data professional should always be learning and stay on top of the trends of his/her respective industry. So, continue to develop your network and keep on looking for professional and educational development opportunities through conferences, bootcamps etc.
Bottom line
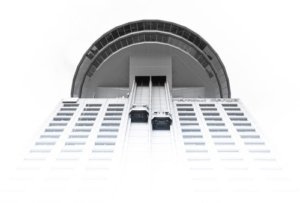
Today, it’s a universal fact that data has become the backbone for almost every industry, in one way or the other. Businesses have moved far from being only focused on their products or services to being data-focused. Even the smallest piece of information these days can bring great value to organizations that can derive a huge amount of insight from it. This has resulted in an exponential increase in the need of professionals who can help the companies accomplish their goals.
The knowledge and insight derived from analyzing data with the help of data science professionals and big data analytics professionals by using the right techniques and tools can help these companies drive product and service innovation. Each of the areas of data science and big data analytics is extremely important to organizations. So, if you’re looking to step into the field of data, you can consider charting your career path for either of these fields based on your preference and abilities.