In the field of data science, projects play a crucial role in developing practical skills and applying theoretical knowledge. However, not all data science projects are alike. A good data science project must meet certain criteria, including relevance, complexity, potential impact, and a process of iteration and improvement.
A significant number of newcomers in data science tend to spend a huge amount of time developing theoretical knowledge and earning certifications alone. While theoretical knowledge is certainly required to become a good data science professional, recruiters don’t put much emphasis on certifications only. Instead, they tend to evaluate the potential of a candidate by going through his or her previous projects.
In this blog post, we will explore the key elements that contribute to a good data science project. We will see how you can develop a strong project idea.
Defining a good project
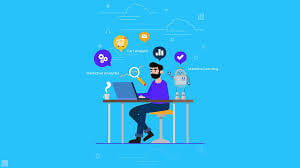
A good data science project should have the following key elements.
Firstly, the project should be relevant to a particular domain or problem area, ideally one that is of personal interest or professional relevance to the practitioner.
The project should also be of an appropriate level of complexity, requiring some level of expertise to complete. On the flip side, it shouldn’t be overwhelming or unmanageable.
Another important consideration is the potential impact of the project. A good data science project should have the potential to make a meaningful contribution to the field or domain, either by generating new insights or informing decision-making processes.
Additionally, a good project should incorporate a process of iteration and improvement, rather than being seen as a one-off activity. This means that the project should provide new opportunities for learning, development, and exploration.
Developing a Data Science Project Idea
In this section, we will explore some tips and techniques to help you develop a good data science project idea that meets the criteria discussed earlier.
Identifying a Relevant Problem or Challenge
The first step in developing a data science project idea is to identify a problem or challenge in your particular domain. This could involve looking at industry trends, examining social issues, or exploring emerging technologies.
Conducting Background Research
Once you have identified a problem or challenge, it’s important to conduct background research and explore available data sources. This might involve gathering data from public datasets or conducting surveys or experiments. By doing so, you can ensure that your project is based on accurate information and insights.
Additionally, data should be cleaned and preprocessed to eliminate any errors or inconsistencies that may hinder the project’s progress.
Considering Different Analytical Techniques and Modeling Approaches
Another key element of developing a data science project idea is to consider different analytical techniques and modeling approaches. This might involve working with machine learning algorithms, using statistical modeling techniques, or exploring new visualization tools. You can try different approaches and determine which one is most effective for your project.
Besides, utilizing the right software and hardware infrastructure can significantly enhance the project’s efficiency and scalability.
Setting Clear Goals and Milestones
To ensure that your data science project stays on track and achieves its objectives, it’s important to set precise objectives and milestones. It is crucial to outline the goals and expectations of the project. You can also break the project down into smaller tasks, establish timelines and track progress along the way.
Measurable Outcomes
To gauge the success of a data science project, it is important to establish measurable outcomes. This involves setting specific performance indicators and benchmarks that can be monitored and evaluated throughout the project.
Conclusion
A good data science project should be relevant, and challenging but not overwhelming. It has the potential to make an impact and involve a process of continuous improvement. It should start with a clear problem statement, use high-quality data sources, apply appropriate analytical techniques, and report findings clearly and concisely.
To develop a data science project idea, one should first identify a relevant problem, do some research, explore different analytical techniques, and set clear goals and milestones. By following these guidelines, aspiring data scientists can create and work on meaningful projects that showcase their skills and offer valuable learning opportunities.
Whether you are a newbie data scientist or a professional, working with real-world data science projects is very important. By following the above tips and strategies, you can develop a data science project idea that is impactful and also aligns with your professional career.
. . .
To learn more about data science, click here and read our another article.