Data Science has emerged one of hot dip topics over the last few years but long before that in 2012, it was declared as “The Sexiest Job of the 21st Century” in an article in Harvard Business Review. If you wonder what had made this domain so hotly discussed today, one reason could be the existence of huge data sets to work upon.
Thanks to hyper-connected people, devices and gadgets, vehicles etc, more data was created in the last two years than in the preceding 5,000 years – combined. Almost everyone and everything is sharing and broadcasting data today. Along with efficient machine learning algorithms and systems to run these algorithms, data scientists can get interesting insights from such large data sets today.
According to Burtch Works Study (2018), an entry-level data scientist’s median starting salary stands at $95,000. For a mid-level data scientist, the median salary is $128,750, but it could rise up to $185,000 in case the person also plays a managerial role. For experienced data science professionals, the median salary stands at $165,000, but those working at the manager-level could earn considerably higher at $250,000. All these make the domain of data science an extremely alluring one.
Before talking about a hot dip topic relevant to data science, it’s important to have a clear idea of what the field is all about. Common people like us may often come across data sets that share no information, no story, and no insight to our untrained eyes. But for a data scientist, the same set of data would open up a world of information. Yes, that’s exactly what a data scientist’s job is – to discover the hidden meaning in seemingly innocuous numbers like these. In other words, you can call a data scientist is someone who pulls out meaning from data. Thus, in a way, a data scientist is a data detective, an investigator with a knack for statistics, or a messenger of meaning. By bringing out interesting stories and insights from inanimate numbers, a data scientist helps businesses spot trends, make informed decisions, and do a lot more.
Before proceeding further, let’s take a simple example of how data science can help you analyze large datasets to make data-driven decisions. Say, you love good Indian food and are searching yelp reviews to find the nearby restaurants where you can satiate your palate with your favorite Indian food. But what happens if you’re in search of a particular type of cuisine and there are several restaurants with the same rating within a small radius? If you have a data science background, you can use it to arrive at a solution. You can consider two things:
- The number of restaurant reviews submitted by a single person of a specific cuisine (Indian food in this case). You can rationalize this parameter by looking at other cuisines’ reviewers, like Italian or Chinese food.
- The reviewer’s apparent ethnicity. In case the reviewer in question has an Indian name, you could deduce that s/he might be of Indian ethnicity, and thus, more knowledgeable about what constitutes good Indian food.
Based on these parameters, you can modify the data gleaned from Yelp. You’re likely to find reviewers with Indian names giving good reviews to some specific restaurants in your vicinity. This way, you’ll be able to arrive at a clear choice of which restaurant you should head out to. So, if you think data science is only for the big corporations, think again. You can even use it to make simpler choices and decisions with greater efficiency similar to the one we’ve talked about in this example.
Now that you’ve got a clear idea of what the field of data science entails, let’s take a look at some hot dip topics relevant to it.
1- Data is an important business asset – much more than you could ever imagine
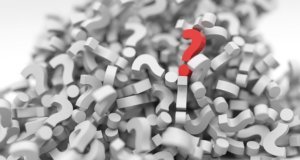
If you don’t believe this, just consider how Facebook’s data breach that affected its 87 million users impacted the 2016 US Elections and ultimately played a huge role in who bagged the coveted post of the US president. So, even if you think your data isn’t useful, many out there think it is cash in their books. In case you haven’t thought about or used the data you collect to the utmost effect, it’s time you do so by engaging a data scientist to spot the insights that such huge pile of data offer for your business.
Let’s take a quick look at the hot dip reason why data is such a valuable asset your business. It lets you understand your website audience types and behaviors better, thus facilitating targeted campaigns to bring in the best results. With proper data analysis, you can understand your audience types better based on their demographics (gender and age group), geographical locations, types of devices they use, other interests etc.
You can even find which customer acquisition channels are working, notice how your website visitors are interacting with/moving around your website, and track your conversion goals (newsletter sign-ups, download of free e-books/reports/whitepapers, buying or short listing products etc). Such insights can help you to fine-tune your business goals as well as marketing and sales campaigns. By knowing what works and what don’t you can create targeted content and strategies to ensure optimum effect and conversions. At the same time, with the help of a data science professional, you can even discover unexploited areas of your business.
Say, you have an online e-commerce store that could expand into a physical store, but where would your brick-and-mortar store be located? An experienced data science professional take a deep dive into your data to reveal the locations that are best aligned with your products, thus empowering your data-driven and informed decision-making process.
2- Data accessibility and proper data collection are just as important
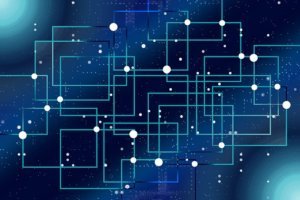
Did you know 74% of firms say they desire to be data-driven, but a mere 29% say they are good at linking analytics to action? Yes, that’s what Forrester (a US-based market research company) found out in 2016. If you fail to easily and quickly get actionable insights from your data, make your company come together around that insight, and are able to take and implement product or business decisions based on it, you aren’t data-driven.
Businesses may not yet consider easy and fast data accessibility across their organization important but it surely encourages and helps in making better decisions. The benefits of training employees in data accessibility include faster iteration, improved efficiency, a competitive advantage, a product-focused approach, and the development of a data-informed culture that leads to better decision-making and actions. In case you’re wondering how you can build a data-informed culture across your company, you can try implementing these basics correctly:
- Everyone is aligned around a solitary core metric that leads toward a common goal
- For everyone, it’s possible to access the data he or she requires easily and quickly
- Everyone gets to know about the relevant insights from their data
To achieve all these, you need a data science professional as your partner. At the same time, you need to acquire relevant information because gathering whatever you (and your employees) can find or lay their hands upon would mean sitting atop a pile of data, most of which is useless. So, when businesses innovate to create connected digital services and devices that generate relevant usage data, they need to focus on acquiring relevant information.
3- AI and its industrialization challenge
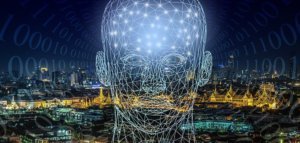
This is another hot dip topic relevant to data science. Artificial Intelligence (AI) has emerged to be as impactful an element as the internet. Machine learning (ML) is an approach to achieve AI and deep learning, which is one of the most advanced forms of ML, is finding a place of importance in several organizations.
Deep learning applications, which are powered by data, are diverse – from natural language processing and image analysis to task automation, and much more. But despite the growing importance of AI as well as approaches and applications connected and relevant to it, the question of AI industrialization challenge has come to the forefront. It has become mandatory for information systems to develop in order to support the diverse uses of artificial intelligence. No wonder why this is a hot dip subject that many are talking about and pondering upon.
When you consider the technical challenges, real-time maintenance, learning, or even scaling of multifaceted algorithms are just a handful of them. To deal with them, the DevOps fundamentals like continuous integration and testing, or containerization need to be applied. The necessary elements required to meet AI industrialization challenges include NoSQL-based new architectures, as well as cloud and data virtualization.
Final words
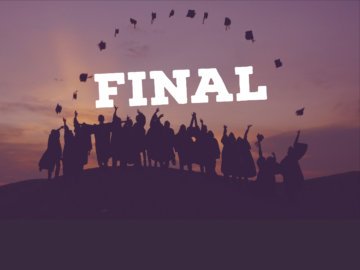
The year of 2019 looks promising for the domain of data science and is likely to be driven much more by data than its predecessors. For businesses, this would mean a growing emphasis on finding actionable meanings and insights from such data by engaging data science professionals. Quite naturally, the hot dip topics these days are revolving around the field of data science and how best the vast amount of data acquired from multiple sources can be put to the best use.
. . .
To learn more about data science, click here and read our another article.