In the very competitive telecommunications industry, retaining customers is essential to long-term success and expansion. However, given the abundance of options and shifting client tastes, telecom companies also have to deal with the problem of customer churn, or the occurrence of consumers terminating their services. To solve this issue, data science has developed into a powerful tool for assessing customer behavior and predicting attrition. Telecom companies may use advanced analytics and machine learning algorithms to identify at-risk customers and implement focused retention strategies. In the end, this strategy increases client satisfaction and business success. The use of data science methods to customer churn analysis in a telecom company will be examined in this paper, with a focus on the consequences for customer experience and retention initiatives.
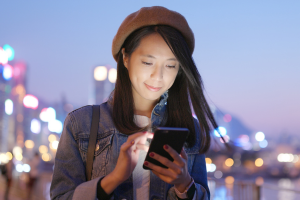
Churn Analysis in the Telecom Industry
Millions of consumers use the telecom operator under investigation for a variety of services, including mobile, internet, and television. It is a major player in the industry. In view of the increasing competition and changing market environment, the company sought to improve its understanding of customer turnover trends and take proactive steps to prevent customer attrition.
Large-scale consumer data collection from several sources, including transaction histories, service usage patterns, demographic data, and customer interactions, was the first step in the customer churn analysis program. Information on previous customer retention campaigns and churn incidences was also gathered in order to compile a sizable dataset for analysis.
Data scientists were able to extract important insights from the unprocessed customer data by using feature engineering. Features such as call time, data use, billing habits, tenure, and customer complaints were developed to capture relevant elements of consumer behavior and interaction.
To predict churn, machine learning models were then developed based on the generated characteristics. A range of classification techniques were employed to build prediction models that may potentially identify customers who were vulnerable to attrition, such as decision trees, ensemble approaches, and logistic regression.
The anticipated churn models were thoroughly trained and verified using historical data. Subsets of the dataset were created for testing and training. The models were trained on past churn situations and evaluated against known outcomes. Recall, accuracy, precision, and F1-score were among the performance metrics used to evaluate how effectively the models predicted churn.
The organization’s operational systems included the projected churn models after they were validated. Because the models are fed real-time data streams from customer interactions and transactions, churn risk can be continually monitored. Thanks to automatic alerts and notifications, the retention team was able to respond quickly when customers showed signs that they might want to quit.
The telecom company saw noteworthy outcomes from the deployment of data-driven churn study. By anticipating client attrition, targeted retention strategies such as loyalty rewards, customized offers, and proactive customer outreach initiatives may be put into place. As a result, churn rates decreased, increasing revenue stability and client retention. Furthermore, by addressing problems and enhancing the customer experience, overall customer satisfaction increased, which promoted advocacy and sustained loyalty.
In conclusion, the case study is a great illustration of how data science has transformed customer churn analysis inside the telecom industry. By using advanced analytics and predictive modeling technologies, telecom companies may deploy targeted retention strategies, get actionable insights into customer behavior, and predict customer attrition with high accuracy. As long as customer expectations stay the same, data-driven approaches to churn control will be crucial for sustaining growth and increasing competitiveness in the dynamic telecom sector.